What do we know about recommendations?
Today it is difficult to imagine any site, service or blog without “maybe you will still be interested”, “read more” or “you might like”…
This is the result of recommender technologies that continue to conquer the world. Over time, their algorithms have improved so much that it seems as if someone is reading our thoughts.
What is a recommender system? This is a complex of algorithms, programs and services, the task of which is to predict what may be of interest to a particular user. Offers are selected based on the study of user behavior.
A simple example: remember the sensational TikTok platform. People can’t take their eyes off their phone screens for hours. After all, they like the content offered and each video is even more addictive.
Let’s try to figure out what the secret of these technologies is and when they became popular.
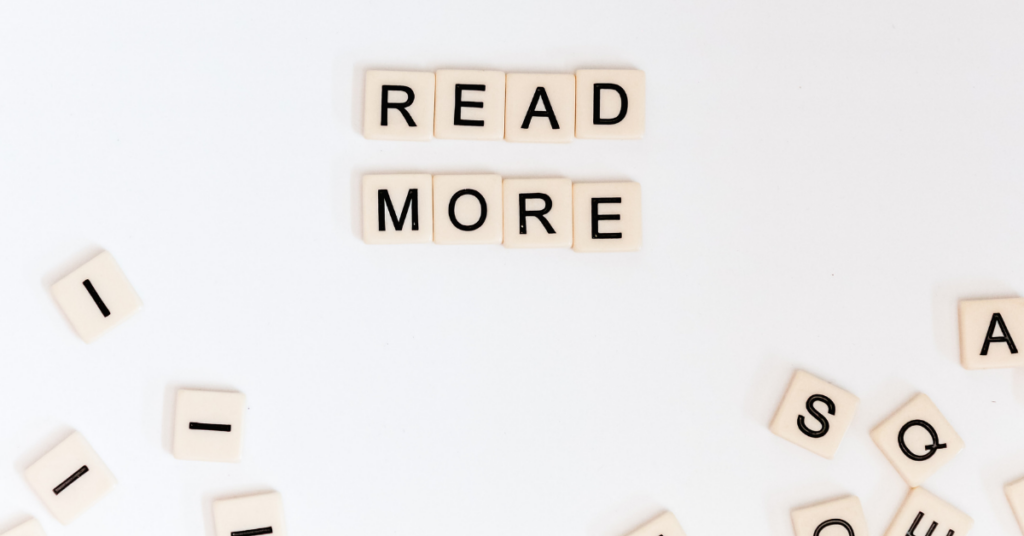
How did it all start?
Until 2006, recommendation technologies were considered niche and were not popular among developers or websites. But the situation was changed by the development, which became known as a result of the Netflix Prize competition and worked from 2006 to 2009. The goal of the project was to improve Netflix’s Cinematch technology, which offered users new films, and the goal was to increase the accuracy of hits by at least 10%.
The Bellkor Pragmatix Chaos team won a $1 million award for a solution that combined 107 different algorithms. Their program improved Cinematch’s accuracy by 10.06%.
Now Netflix is a giant of media services, and in the early 2000s the company provided movie rentals on VHS and DVD. It was important for them to guess the preferences of users. Thus, after the competition, interest in the recommendations grew.
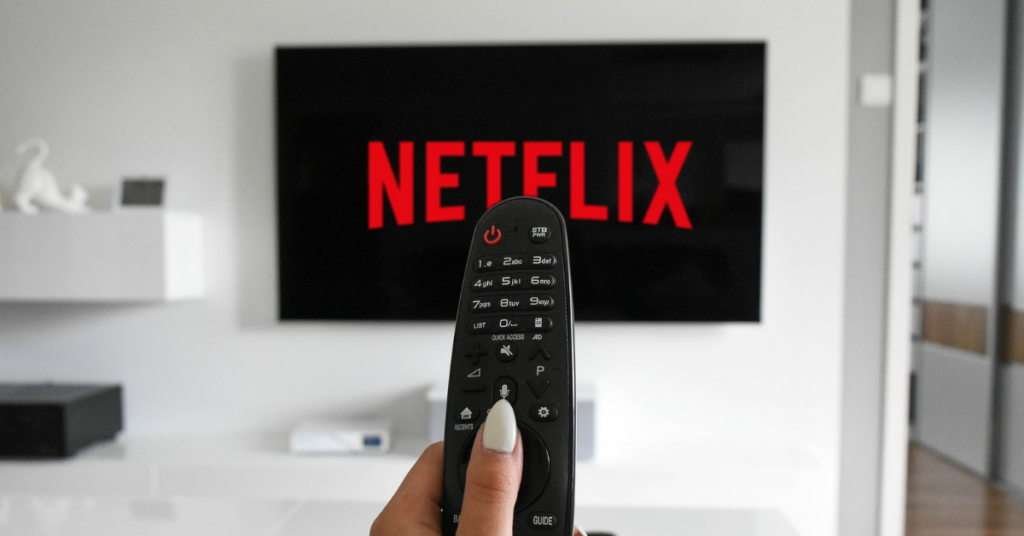
How recommender systems work
Content-based filtering
The basis of this method is the browsing history, through which the content is offered. For example, a housewife who has logged into the Cooking blog and looked at several recipes will be advised similar materials on the same site, where she can learn more useful information.
Collaborative filtering
In this case, recommendations are issued based on the behavioral characteristics of the person or group. A prime example of such a method would be the Spotify platform. It takes into account not only the general client interests and preferences, but also the temporal context. For example, a lover of heavy rock music listens to it in the morning or on the way to work, and in the evening puts the children to bed to gentle musical compositions of a completely different genre.
Knowledge-based filtering
This method is the most accurate, because it represents objects not only based on their characteristics, but also taking into account rules and restrictions. For example, it makes no sense to offer to buy a product that is not on sale.
Hybrid filtering
They combine different approaches. Using them, you can get rid of most of the shortcomings of other methods and provide the user with the most accurate advice.
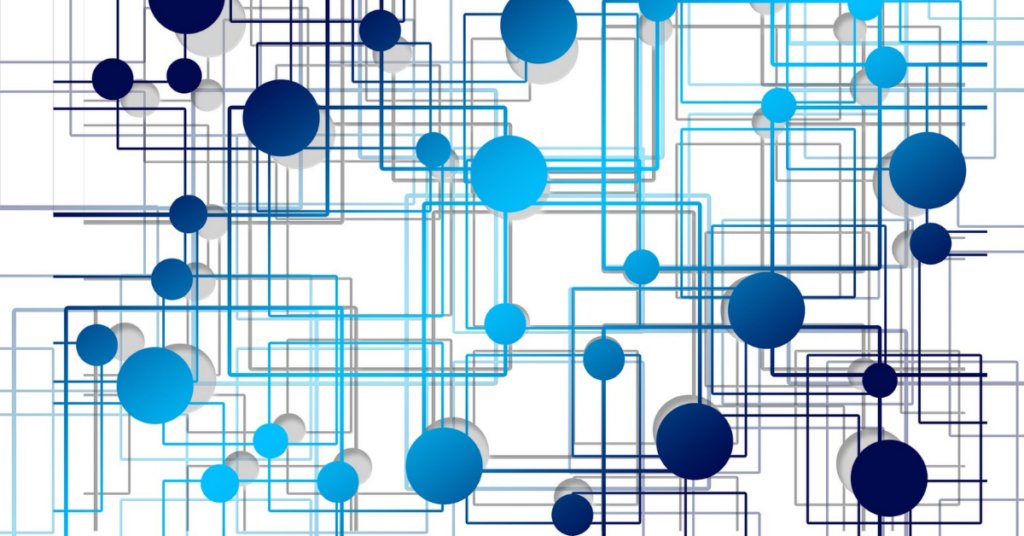
How useful are such systems?
Users love it when all the necessary information is in front of their eyes and there is no need to make extra efforts to search. Everyone likes it when their interests are taken into account and all expectations are met. Recommendation blocks are perceived by users as advice and are not associated with annoying ads. A personalized approach helps to position the reader to the resource, as well as encourage him to take action.
“Advice is more than a service”.
– A. Dumas-father
As for entrepreneurs, such technologies open up new opportunities for them. They allow the site to offer its articles to users. The better the recommendations, the more likely it is that the user will be interested and stay on the site. Accordingly, the effectiveness of the site and income increase.
If you are the owner of a web resource and do not know where to start, we advise you to turn to the Phoenix Native platform. Its effectiveness lies in the fact that it can adapt to any user and offer him relevant content. The system conducts a deep analysis of user behavior, processes data and generates offers with maximum accuracy.